Robotic Process Automation and Machine Learning
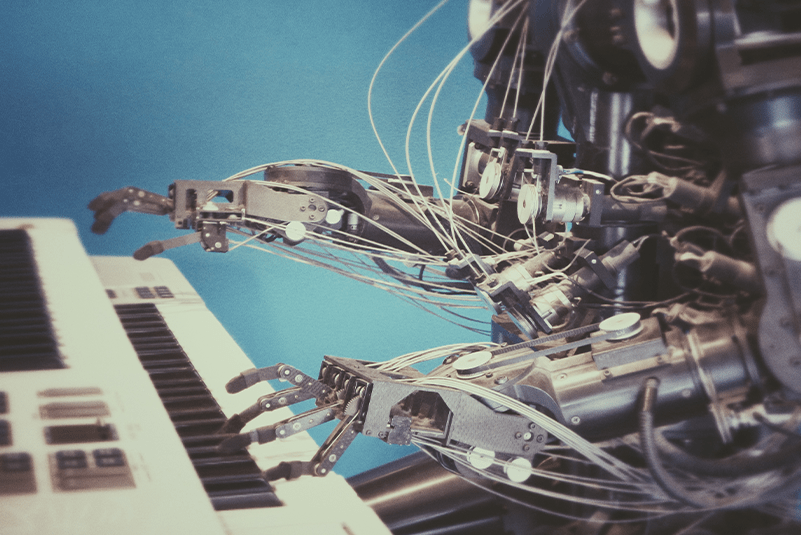
Machine Learning
Machine learning and robotic process automation seem to have a bright future ahead of it. The term machine learning was not known by people five years back. However, it has become prominent among people in today’s world. It is widely being used for a variety of applications in today’s world as well. OneBusiness a cloud-based software is a perfect platform for machine learning and robotic process automation.
The capabilities of machine learning
With the help of machine learning, it is possible to predict the behaviour of dynamic systems that exist in the real world. For example, the way how people will work in a crowdsourced project can readily be determined along with the assistance of machine learning. Likewise, it is also possible to determine how a marketing campaign would deliver positive results for the people.
Data related to the behaviour of these systems is generated by live agents. The systems will keep on analyzing behavioural data as well. As a result, the system will tend to learn along with time. In the meantime, agents will be observing all the changes that take place within the system as well. Then they will respond to the specific changes through their behaviours. This is the reason why behavioural data evolves along with time. They are not independently and identically distributed. As a result, the theories behind machine learning have come across some significant theoretical challenges as well.
Robotic process automation and machine learning have ensured a brighter future for Cloud ERP solutions as well. The functionality of cloud ERP software can easily be enhanced with the help of these technologies. Machine learning on cloud ERP can benefit businesses with their planning. Hence, businesses can quickly improve their processes along with time. You can visit onebusinesserp.in to get more information on this.
Markov Chain in Random Environments
These theoretical challenges can easily be mitigated along with the assistance of Markov Chain in Random Environments. Markov Chain in Random Environments, which is also known as MCRE can be used effectively to determine behavioural data. Also, it can conduct a generalization analysis of the algorithms used in machine learning.
MCRE is associated with a one-step transition. Due to this reason, the outcome you get out of it is dependent on the random environment as well as the previous state. That’s why traditional techniques cannot be applied in this situation. To address this issue, MCRE should be transformed into a time-homogeneous Markov chain, which is located in a higher dimension. Even though it comes along with a higher number of variables, the platform will be regular, and people will find it as an easy task to deal with it. When the time is sent to infinity, the Markov chain will converge. This can be used to prove generalization with machine learning algorithms when they are used along with data generated via Markov chain. Data will be dependent on both covering numbers and Markovian parameters. Hence, it is the most effective generalisation analysis method, which can be used to model dynamic systems in the real world.
A considerable percentage of data available for consumption in today’s world are generated by interactive systems. In other words, no humans are involved in the process of data generation. To ensure a reliable mechanism, it is essential to determine the agent behaviours beforehand. If there is a possibility to predict agent behaviours effectively, the platform will be able to optimize the performance. As a result, the process of attracting more agents to the system can be made easy.
Applications of agent-based modelling
Agent-based modelling and simulation, which is also known as ABMS can effectively be used to model systems that are made out of interacting, autonomous and individual agents. The applications of ABMS span across a diverse range of scenarios. They include determining the success of promotional campaigns, identifying the chains within the stock market and projecting the upcoming requirements of the healthcare systems. Back in the day, agent-based modelling was used to determine the spread of AIDS. This can be considered as one of the initial applications of the model. After that, it was used for many other successful applications. These applications became more prominent after the 1990s. These applications include economics, public policy, and management of ecosystems, insurgency, crime proliferation, tax evasion, technology adaptation, traffic patterns, crowd dynamics and stock market behaviour. It is also possible to figure out some prominent applications of agent-based modelling related to human learning and human cognition.
Complex adaptive systems can easily be modelled along with the usage of agent-based modelling. It is known as the most suitable approach to proceed with modelling. It can effectively accommodate complex dynamics and structures. On the other hand, it is possible to move with the construction of models without having a clear understanding of the global interdependencies. Moreover, agent-based modelling has been identified as the most suitable approach available to move forward, when modelling a system that indicates adaptive behaviour.
It is possible to find agent-based systems being used in a large number of systems located across the world. To manage this kind of a system effectively, it is essential to have a clear idea about the individual components and the way how they interact with each other. These come along with their responsibilities and rules as well.
Agent-based models recently gained a lot of attention to water-related sciences. The demand available for water can effectively be modelled in this manner. As a result, it is possible to explore several other dimensions as well. Risk management during a disaster can be considered as a perfect example to prove the fact mentioned above. With this model, it was possible to come up with effective flood management procedures as well. Moreover, it can be used on a grander scale for Tsunami evacuation as well.
When modelling a system through agent-based models, it is essential to specify the exact relationships that exist between individual agents. The interaction agents should be located within the environment. Otherwise, there is no possibility to establish the links between them. Moreover, a variety of methods are also available to build connections as well. Goal-directed relationships and simple reactive relationships are to name a few. Also, the specific environment should be determined as well. This can facilitate the interactions that take place within the system.
BENEFITS
Several benefits are linked with agent-based models. These benefits have tempted people to use an agent-based model in various applications. After the creation of an agent-based model, it is possible to explore new dimensions as well. The applications will evolve in the future as well. Hence, it will be possible to find a large number of prominent applications of agent-based model shortly.
On the other hand, multi-agent systems and cloud-based software are being used to model and simulate complex systems, which span across various contexts. They range across biological systems and social systems. It can be considered one of the most successful and productive forms of agent-based modelling. With multi-agent systems, it is also possible to stimulate crowd and pedestrian dynamics.
From one of the recently conducted experiments, multi-agent systems were used to manage the carbon footprint within a corporate environment. This was done along with the participation of a heterogeneous system. Cognitive Agent-based Computing played a prominent role in here. Through this model, it is possible to depict an autonomous system.